This article was originally published by DAM supplier, Tenovos as a chapter in the Guide to Data in Digital Asset Management.
Data is the foundation of your DAM. From the way users find content to how you calculate content performance and ROI, it all depends on the data foundation you’ve built.
In this guide you’ll learn:
- How to think about data as a foundational element of your DAM
- The power of keywords in digital asset management
- How data maturity can lead to increased ROI
- AI, machine learning and the future of DAM
AI, Machine Learning DAMs, & The Future of Data
The future of Digital Asset Management is here!
Data is the lifeblood of any organization, and metadata is what creates value from otherwise unstructured files in DAM. It’s what we use to make decisions, understand our customers and improve our products. It’s how DAM users search and find the assets they need and how a DAM can automatically deliver the right asset to the right channel. Data and metadata can also make assets more accessible to customers and improve the overall customer experience.
However, data is costly to enter, maintain, and update to the high standard of quality and accuracy necessary to power DAM use cases such as end-to-end content lifecycles, brand management, omnichannel delivery, and personalized customer experiences. As digital assets continue to proliferate, it’s becoming more and more difficult for humans to manage all this data.
To maximize the value and return on investment from both our DAM technology and the creative content that lives in our DAM, many organizations are now exploring artificial intelligence (AI) as an innovative approach to enriching metadata and data about our digital assets. While human intervention and moderation is still necessary, AI technologies can add metadata to assets at a greater speed and with more accuracy than humans could achieve on their own, enabling companies to scale their content production and delivery while simultaneously increasing staff efficiency.
What is artificial intelligence, and how can it be applied to improve metadata in digital asset management? Simply put, AI is a process of making a computer system “smart” – that is, able to learn complex concepts and carry out complex tasks. In the context of digital asset management, this means that AI can be used to automate tasks such as asset recognition and tagging, metadata entry, categorization, and more. This can save time and improve accuracy when managing digital assets.
Before we jump in, let’s define a few terms that fall under the umbrella of AI, including machine learning, deep learning, and computer vision.
Machine learning is a subset of AI that focuses on the ability of machines to learn from data and improve their performance over time. Machine learning is particularly useful for brands who want to “teach” AI to understand brand-specific, proprietary concepts, categories, and visual cues.
Deep learning is a subset of machine learning that uses algorithms to model high-level abstractions in data. In the context of digital asset management, a combination of machine learning and deep learning can be applied to complex tasks such as:
- Computer vision: AI that can process and interpret digital images, video, and other visual content
- brand-specific asset recognition and classification
- facial recognition
- logo recognition
- landmark detection
- object localisation
- text recognition
- crop hint detection
- explicit content detection
- visual search
- visual similarity search
- Natural language processing
- Translation
- Pixel restoration
- Text generation
- Colorization of black & white images
- Sound addition to silent films
- asset recommendations
- Speech recognition
How AI Is Changing DAM As We Know It
Due the spectrum of capabilities offered by artificial intelligence technologies, AI in DAM may be a game-changer across the full range of DAM use cases, from:
- Asset search & discoverability
- Asset management
- Data-driven decision making and insights into asset effectiveness
- Creative asset creation and scaling production
- Brand management
- Customer experience and personalization
Artificial intelligence in DAM has made significant progress in recent years, with organizations that are able to fully capitalize on it seeing measurable results. Some of the world’s top brands, across all sectors and industries, are already using AI to improve their data and digital asset metadata.
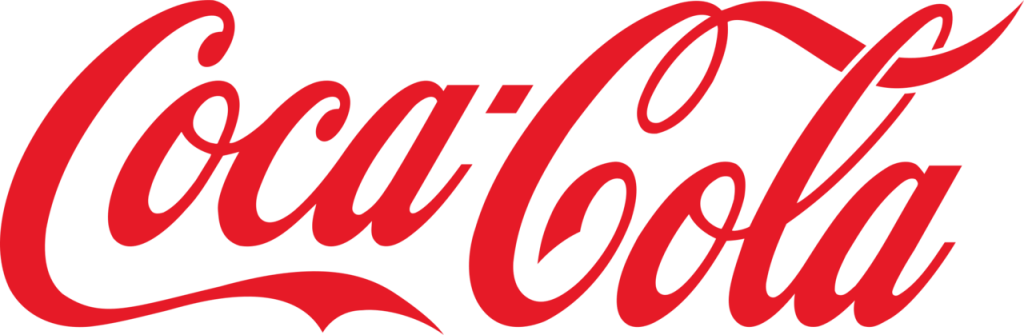
Companies like Coca-Cola use AI to automatically tag and categorize images in their DAM systems to save time and ensure that assets are properly organized and easy to find.
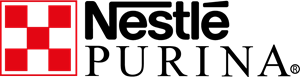
Product retailers and CPGs, like L’Oréal and Nestle Purina, can use AI to develop bespoke brand-identification systems that can automatically generate product descriptions and metadata, even applying brand tone of voice. Such applications of AI improve the accuracy of the company’s product information and make the data more accessible to customers.
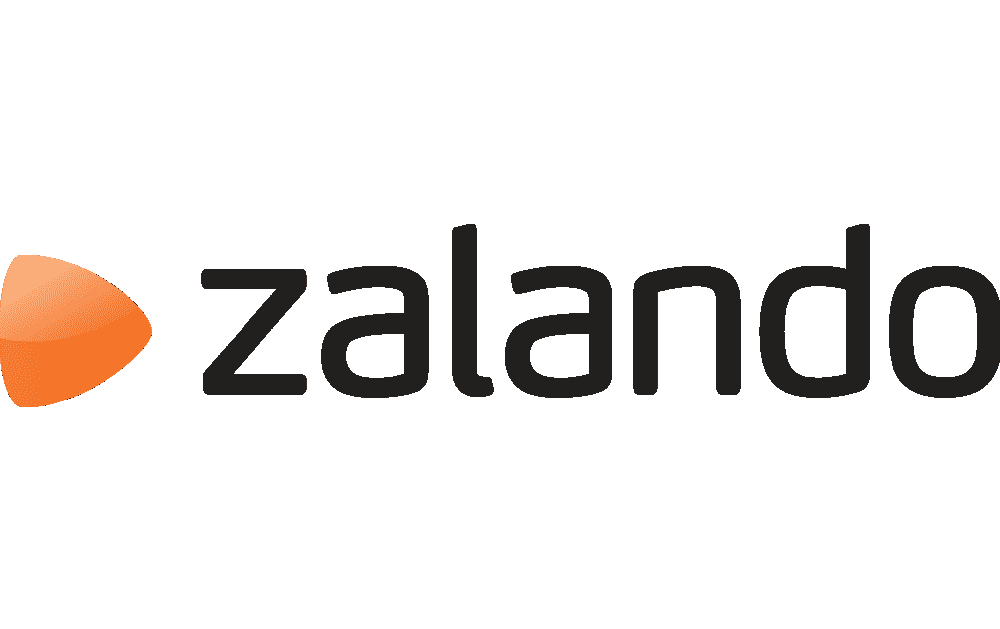
In the fashion and eCommerce industries, AI is being used in DAM systems as a recommendation engine for similar or complementary fashion goods to up-sell customers and personalize advertisements, provide information to designers about current fashion trends, manage contracts and rights with models, and make content containing AI models and 3D AI clothing searchable.
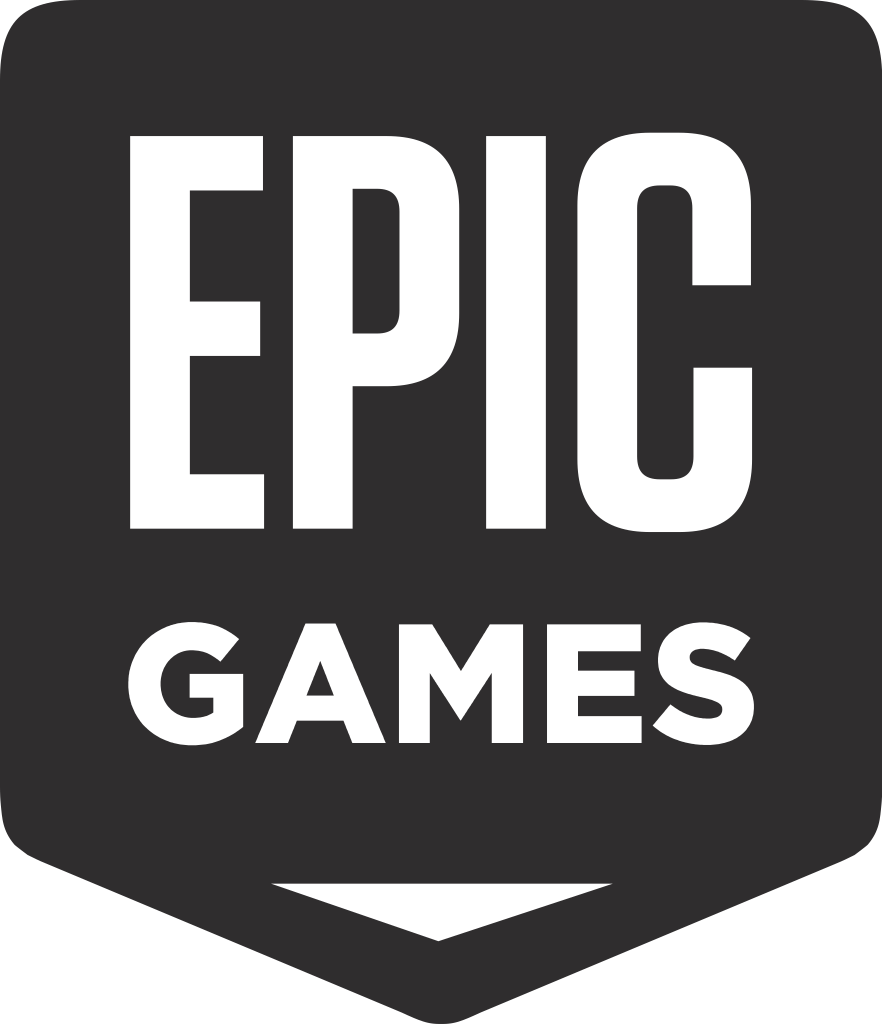
In the video gaming industry, AI can automatically identify and track the behaviors of characters in stills and videos, recognize actions such as explosions or a dancing Fortnite character in player screen recordings, moderate content for appropriateness, and automatically clip and generate montage reels from a player’s game.
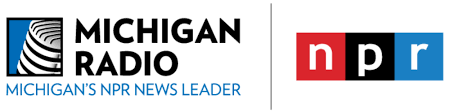
News media companies, as well as local newsrooms, use AI technology for newsgathering from social media and visual search of photo sets, as well as news production including automatic transcription from videos and audio, text summarization from videos, and video clipping. Some AI tools can scour all of the videos and audio in your DAM and automatically apply speech-to-text transcriptions, closed captions, and automatically clip relevant sections based on your search.
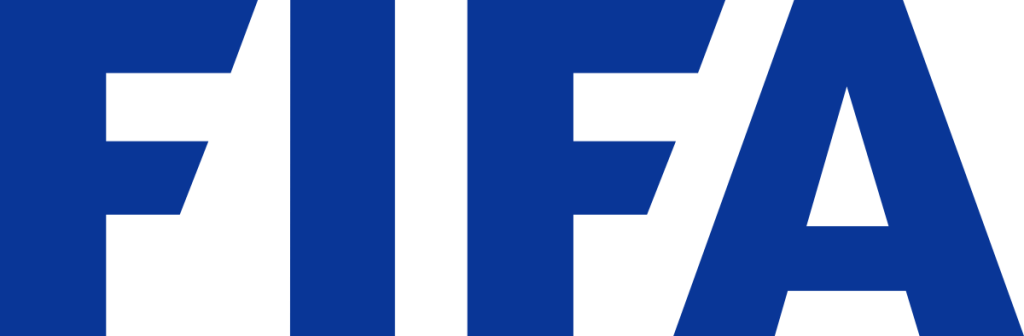
In the sports industry we see AI used for applications ranging from refereeing, building personalized training and diet plans for athletes, and ticketing for sports venues. And when it comes to data for digital assets in sports, AI computer vision can combine facial, logo, and jersey recognition to accurately identify players, coaches, and sports highlights within seconds. During live televised sports events, this level of speed and sophistication can enable marketing teams to post key moments to social media almost immediately and can support commentators who need to quickly find and refer to slow-motion replays. Not to mention, data collected by AI technologies using sports analysis techniques to review hundreds of hours of footage can provide vital information for sports predictions and athletic performance insights.
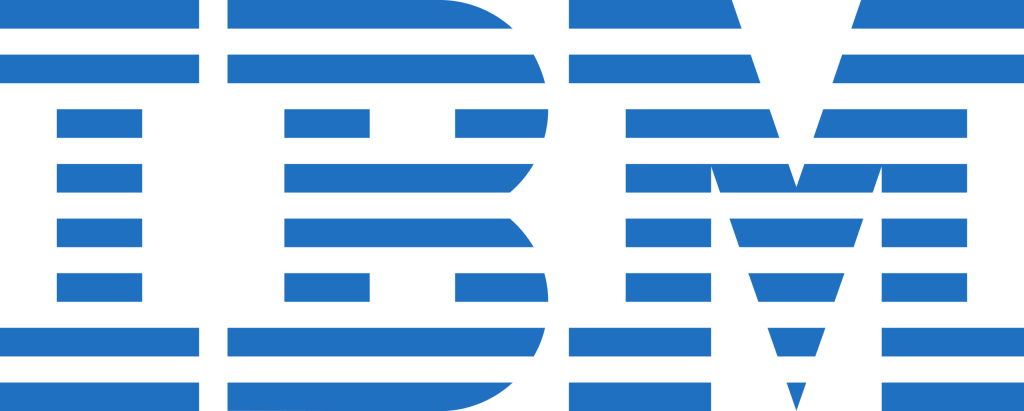
AI is not just for large corporations. AI in DAM is also prevalent in scientific and cultural heritage communities. The healthcare and medical industry can use AI in DAM to relieve doctors and nurses from tedious manual tasks by automatically classifying types of exams (X-Ray or MRI) and organ groups shown on medical images (lungs or liver), with a success rate that far exceeds human ability. Cultural heritage organizations have further utilized AI in DAM to delve into vast, unstructured digital archives to unlock new revenue streams, aid discoverability, and identify biases and harmful language in cataloging to apply reparative metadata principles.
With AI-powered DAM processes, such as searching for assets, delivering optimized assets, and archiving assets, marketing teams can work smarter, not harder. AI can be a powerful tool in digital asset management. By automating repetitive tasks and improving accuracy, AI can help you save time and maximize your marketing efforts.
Turbo Charge Your Creative Asset Lifecycle With AI
Repetitive, manual tasks are the bane of our workdays and the disrupter of creative thinking. A study by Hubspot from 2015 shows that the average marketer spends about 16 hours per week on routine tasks. Logically, then, we know that we can give our marketing teams up to 35% of their workweek back for higher-level projects if we find ways to relieve repetitive, tedious tasks, something that AI technologies are engineered to do.
In digitally mature companies, a well-integrated DAM within a wider marketing technology ecosystem, aided by AI and automation, can transform creative asset workflows into streamlined, efficient, data-driven processes. In an integrated ecosystem where digital assets flow through or gather data from foundational tools such as DAM, PIM, ERP, MRM, workflow tools, MDM, data and BI tools, through to engagement services and channels such as CMS, eCommerce, CRM/CDP, social media platforms, marketing automation, and email marketing tools, you can start to track data across the entire digital asset workflow.
This data can then be used to make informed decisions on everything from what type of assets to create, how to better target your audience, when is the best time to release new content, and where your marketing efforts are most effective. AI in DAM can help you not only automate processes but also glean actionable insights from your digital asset data, making your entire marketing operations more efficient, data-driven, and agile.

With the help of DAM, AI, and a group of skilled data analysts, we can track, visualize, and analyze valuable data along the digital asset workflow to extract highly sought after insights such as:
DAM User Interactions
- Which users interact with the DAM the most across the asset lifecycle?
- Which resources have the skillsets and experience to be matched with this next campaign?
- How long does the average digital asset end-to-end workflow take? Where are the bottlenecks and how can we improve the process?
Digital Asset Metadata
- How many assets will expire in the next month? Is it more cost effective to re-license these assets or produce them in-house?
- Where is the asset of the woman with the hat sipping a cocktail?
- The asset I want to use has been overused, which assets are similar but have been used less than 10 times and never on the website?
Digital Asset Lifecycle
- Which individuals worked on this asset?
- How much was spent on it? What was the total cost of production?
- How quickly was this asset delivered to the customer?
- Where has it been used?
- When does the asset expire and when do we need to take it down from our channels?
Effectiveness Data
- How effective was this campaign?
- How effective was this specific piece of content?
- How well did this image of someone using our products perform in comparison to a product shot?
- How do we measure the impact of our assets?
- Can we understand WHAT is working? And WHY?
- Which content is most effective for each audience/region/market?
- Which variant is most effective? Which is least effective?
- Can we save money on production by focusing the marketing budget on the most effective content?
- Which channels and content have the greatest impact on revenue?
Ultimately, CMOs and organizations want to know which marketing tactics are contributing to conversations and sales revenue. Marketing attribution programs can only work if data is gathered and normalized across the full creative content workflow, which includes DAM and a myriad of data from all platforms across the lifecycle.
With all of this data at our fingertips, we can start to make more informed decisions about our digital asset strategy, saving time and money while making our marketing efforts more efficient and effective. So if you’re feeling bogged down by tedious tasks or struggling to make sense of your digital asset data, consider enlisting the help of a DAM system with AI capabilities to make your life a little easier. Trust me, your future self will thank you.
Things To Consider Before Getting Started With AI in DAM
By automating tasks, increasing efficiency, and improving accuracy, AI can be a powerful tool for enriching data and metadata in digital asset management. However, it’s foolish to believe that AI is a magic wand that will simply apply the correct metadata that humans forgot to enter, automatically deliver the assets to us by reading our minds, or provide answers and insights into the effectiveness of our assets.
On the contrary, AI technologies require careful consideration, selection, strategy, and ongoing management, resourcing and funding.
As DAM systems increasingly incorporate AI, businesses are asking themselves how they can use AI to improve their creative processes. Before you dive into AI to enrich the data and metadata on your digital assets, here are five tips on how to get started.
1. Build a shared strategy and vision: Investing in AI can be costly and without a clear strategy and vision for the purpose of using AI, businesses can quickly run down the budget and be left with little return on investment (ROI). Before investing time, resources, and money in a problem that does not exist, an organization should consider the issue it is trying to solve, why AI technology is the right solution to the problem, and what the organization plans to achieve.
2. Choose wisely and test before you buy: Not all AI tools are created equal and there is no one-size-fits-all tool. That’s because there are many types of AI technologies available and each one is specialized to help you solve a different problem. For example, while you may be able to train an AI tool to identify the difference between cereal and dog food in your image assets, you will need to train a different AI technology to identify the executive speaking in your corporate videos. Make sure to test out different AI tools before making a purchase to find the one that fits your needs. Once you’ve decided on the AI tool that is best suited to helping you solve a challenge, we encourage you to speak with your DAM provider to consider integration possibilities.
3. Start small and simple: Don’t try to use AI for everything at once. Begin by identifying specific tasks within your digital asset management system that can be automated or improved with the help of AI. And apply AI to simple tasks before exploring complex tasks. Start by automating simple tasks that can save you time and improve accuracy.
4. Be patient as your AI grows up: Depending on the complexity of the tasks which you want the AI tool to accomplish, you will need to invest time, resources, and clean datasets to “train” machine learning and deep learning technologies. It takes time to train AI tools and to feed them the correct data. Working with a data scientist can help your organizations to feed clean, accurate, unbiased, and comprehensive training assets to your AI tools and continuously improve the outputs from your preferred AI technologies. Don’t expect AI to be perfect from the start.
5. Educate your team: The more your team knows about how to use AI tools, the more productive they will be. Make sure to provide adequate training on how to use these tools in combination with your DAM. Collaborating with robots can be a significant change in the way people are working and having a change management resource and plan to support user adoption is key to your success.
By taking the time to consider these factors upfront, you can set yourself up for success in using AI to improve your creative processes.
What Does the Future of AI in DAM Look Like?
Every major sector and industry has been affected by artificial intelligence in one way or another, but the digital asset management marketplace is still in its infancy when it comes to exploring the applications of AI. However, some innovative DAM providers are already experimenting with advanced AI applications to address common issues in managing huge volumes of data and metadata relating to their creative content.
There is a significant amount of untapped potential in the future for vendors and end-users of AI in DAM, especially around metadata enrichment, asset discoverability and accessibility, creative content production, content personalization at scale, workflow automation, and analytics to power content, creative, and marketing functions.
As more industries and vendors trial new technologies and share their successes with the community, we look toward a future where we can realize the full value and potential of the powerful combination of AI and DAM.
Want to learn more? Listen to the podcast discussion about AI & The future of data in DAM with Kristina Huddart and Tenovos here: https://tenovos.com/resources/digital-assets-data/dam-podcast/